September 2024 Issue Index
Delivering best scheduling outcomes
Tackling mine production scheduling with its inherent uncertainty, ambiguity and changing constraints is an ideal challenge for evolutionary computing.
Evolutionary computing is an approach to problem solving that takes inspiration from biology and the way populations of organisms adapt to their environment. By maintaining a population of different sub-optimal solutions to a problem, a program can evolve the solutions over time to become more optimal.
The machine learns how to solve a problem by itself, aligning evolutionary computing as a branch of artificial intelligence. Software engineers choose evolutionary computation for optimisation problems that are too difficult to solve easily—because the best answer is either ambiguous, ever-changing or difficult to find.
Consider the scenario for a mine with variable grade estimations at different locations. Calculating the best way to mine the ore to maximise financial returns is complex for all but the simplest orebody.
The answer is ambiguous because only an estimate of the ore is known, ever-changing because commodity prices fluctuate, and difficult to find because a huge amount of data is required to specify the problem in detail. Characteristics of the mining fleet and processing plant must be included as well as knowledge of the orebody and the non-linear relationships between the systems.
When a deleterious element exceeds a threshold concentration in the processing plant it causes the recovery of ore to drop sharply. However, recovery is barely affected if this element is below the concentration by an equivalent amount. The response of the plant to the concentration is non-linear.
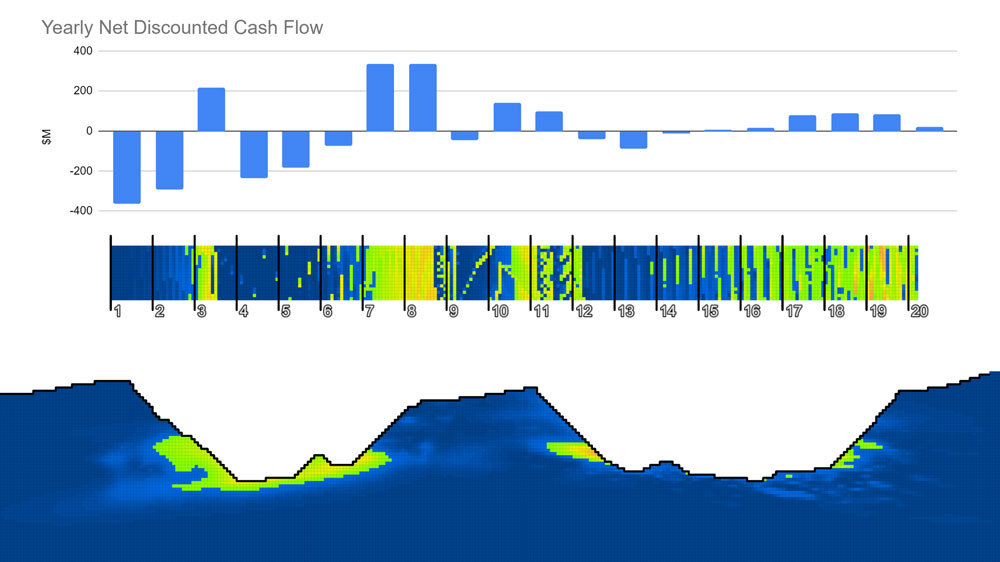
Optimum solutions
Miners need secure ways to understand the true nature of their data and the problems they are trying to solve. Maptek™ solutions enable decision making informed by actual data, not based on linear trends or gut feel.
Maptek applies evolutionary computation to tackle the mine production scheduling problem the way it should be tackled—with its inherent uncertainty, ambiguity and changing constraints. Other software can provide precise formulations of a brittle model—the solution looks good on paper but cannot be realised with real data.
Economic forecasting is extremely difficult. The more tools you have to rapidly compute ‘what if’ scenarios, the more assured you can be that your plans will reflect the most probable scenario or that you have assessed the downside risks.
This is not always the same as finding the optimum solution, which explores many solutions simultaneously, evolving towards optimality.
Like most businesses, miners typically underestimate the value to be gained of using already collected data in a smarter way. Integrating a continuous flow of the latest information can revolutionise the decision realm.
In a dynamic environment, when goals suddenly change, teams can be overwhelmed. Mine scheduling will never be free of surprises but when they appear, evolutionary computation will have unlocked understanding ahead of time, allowing an alternative near-optimal course of action to be chosen.
Maptek provides the automation tools and orchestration platform to swap focus from when and how to rework a mine plan, towards continuous re-evaluation of the schedule using live data.
Mining is overflowing with optimisation problems that are challenging to tackle. A data driven approach empowers miners to apply the knowledge gained across the mining value chain for better decision making.
- Evolutionary computing is the most effective approach for solving complex optimisation problems
- Computing ‘what if’ scenarios assures miners that their plans will reflect the most probable scenario and/or help assess the downside risk
- Automation and orchestration can switch focus from when and how to rework a mine plan, towards continuous re-evaluation of the schedule using live data