Data driven modelling in a production environment
DomainMCF can change the way an operating mine uses geological and geotechnical models to keep information up to date in a production environment.
A trial of Maptek DomainMCF to accelerate ground control model creation has opened the door for Sibanye-Stillwater to apply machine learning to other aspects of its operations.
DomainMCF is a simple application capable of absorbing very large datasets. Once the machine learning model has been trained on a dataset, it can generate a predicted domain block model very quickly. Geologists have more time to look for significant features and trends that may impact safety, productivity and profitability.
DomainMCF allows a more timely and practical use of the valuable investment in data collection and storage.
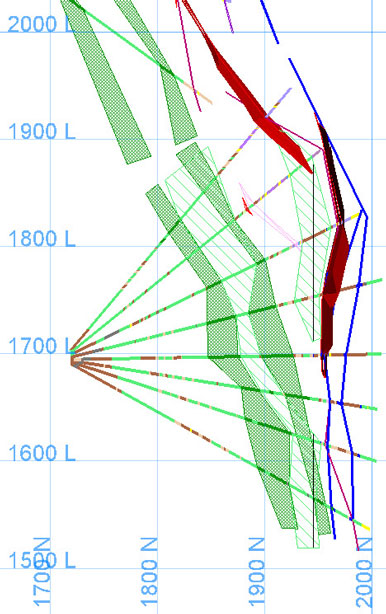
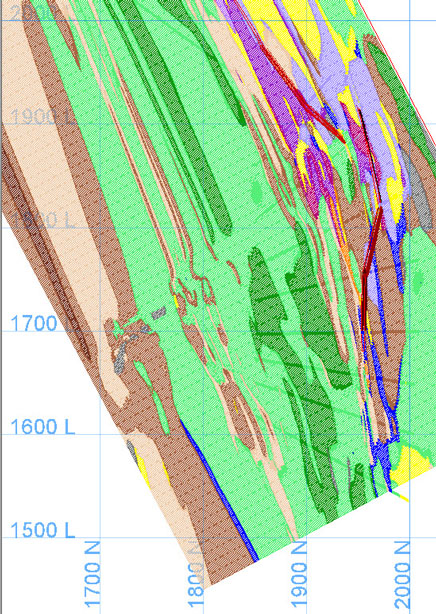
Digitised cross-section (left) and DomainMCF version (right)
Stillwater and East Boulder are shallow to intermediate level underground PGM (platinum group metals) mines in south-central Montana. The two principal mining methods are mechanised ramp and fill, and sub-level extraction by longhole open stoping with hydraulic backfilling. Efforts to expand the production output are ongoing.
During 2020, Maptek began working with Sibanye-Stillwater to evaluate whether DomainMCF was a good fit for their geological modelling work. The focus for experienced Senior Rock Mechanics Engineer, Gretchen Moore was harnessing data for ground control risk evaluation and predictive modelling.
The Stillwater igneous complex has been recognised as a metalliferous resource since the late 1800s. Copper, nickel and chromium were identified in surface outcrops and the chromium was mined as a strategic resource in the 1930s.
The platinum group orebody lies within a horizon referred to as the J-M Reef, a world class stratiform magmatic reef-type deposit.
Drilling and modelling
Production definition diamond drilling programs involve primary axis drifts being driven 200 to 300 feet south of and in parallel to the J-M reef. These drifts are 300 to 400 feet apart vertically, and are driven out in front of the area of high concentration of existing diamond drillhole information. At every 600 to 1200 feet of primary drift, Sibanye-Stillwater performs campaign diamond drilling of the reef, which targets a 50-foot spaced grid through the narrow deposit.
Holes are logged in their entirety for lithology, structure, alteration and mineralisation, and selectively for geotechnical data within about 30 feet north and south of the mineralised zone.
‘Drilling scheduled during 2020 involved the geologists logging upwards of 800,000 feet of diamond drill core – to say this is a lot of data is an understatement’, said Moore.
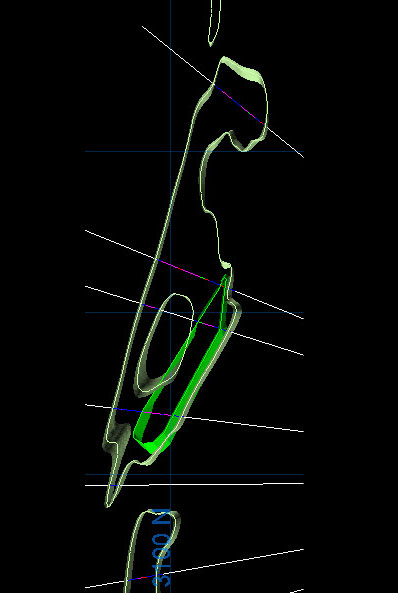
The development geologists traditionally digitise 2D cross section interpretations. This group is also responsible for designing drill programs, logging and interpretation.
Reserve and resource geologists update interpretations of the mineralised zone, creating the minimum mining model that is used to design stoping areas and calculate reserves.
The geotechnical model is built using composited geotechnical or lithologic data to predict the anticipated ground types, and the ground support required in all development and production headings.
‘Historically, ground-type models were only built in the areas we planned to mine, and it was fairly labour intensive using the ore triangulation that the geologists build for reserve modelling,’ Moore said.
The main purpose of the machine learning trial was to estimate the geotechnical variables into a block model. Where the geotechnical data is not collected, gaps are left in the ground-type model.
DomainMCF
Moore researched software to expand the use of geotechnical models and saw the potential value in Maptek DomainMCF.
‘It’s not just faster block modelling – updating models in minutes, not days. It also enables us to include the latest information in planning and forecasting,’ Moore said.
Sibanye-Stillwater data exists in a standardised format, so little data manipulation was required for a successful test modelling run.
Data input into DomainMCF consisted of text lithology logging presented as a numeric rock code representing the 21 lithological units to be modelled. The first test model took 2 hours and 15 minutes to generate.
The geologists were excited to see how DomainMCF modelled the thin problematic rock types and structures that are important for predicting ground control conditions in the mine.
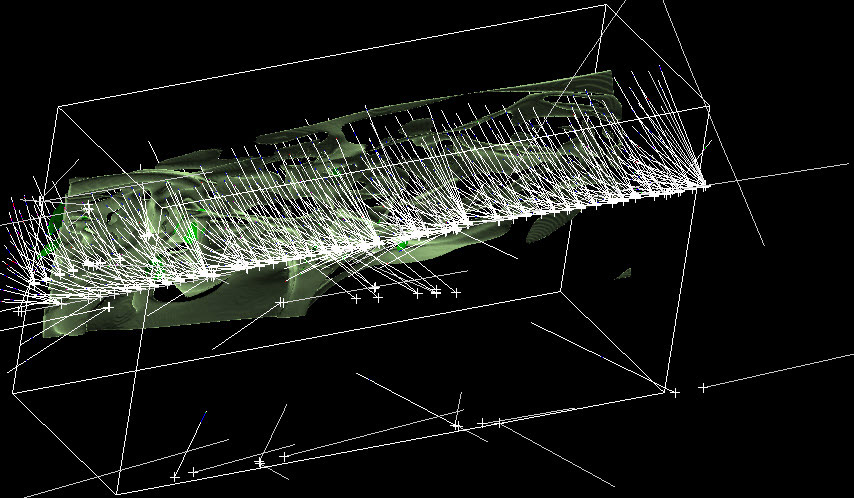
The development geology team soon realised they could spend more time on doing actual geological interpretation and structural modelling rather than on the manual drafting process.
DomainMCF was then tested on the mineralised domain, for comparison with the current explicit modelling workflow. The resource geology team will supervise ongoing reconciliation and proving tests during 2021 and 2022.
For the geotechnical ground control models, the DomainMCF test models showed significant improvements in accuracy, time to delivery and effectiveness, to guide geologists and mine planners in ground support requirements.
DomainMCF software usage costs are based solely on the actual compute time it takes to generate the geological domain models. Maptek worked with Sibanye-Stillwater staff to extrapolate the expected computing time from early demonstration models, creating a budget usage estimate for the entire mine.
When considering the ease of use for DomainMCF, Moore stated that, ‘Input and setup are quick, and once we dialled in some of the sensitivities, I could set a model up to run in less than an hour. The level of detail is far greater than could ever be achieved through manual digitisation efforts in any kind of a timely fashion.’
Sibanye-Stillwater geologists and engineers are keen to see the application and impact to short and mid term planning activities as testing and increased utilisation of the Domain MCF models progress.
Thanks to
Gretchen Moore
Senior Rock Mechanics Engineer
Sibanye-Stillwater Mine
- Sibanye-Stillwater trialled the effectiveness of machine learning to harness data for ground control risk evaluation and predictive modelling
- DomainMCF test models showed significant improvements in accuracy, time to delivery and effectiveness for guiding geologists and mine planners
- The speed of model generation with the machine learning approach allows Sibanye-Stillwater to include the latest information in planning and forecasting in a production environment