Unlocking complex resources
Maptek applies advanced techniques to provide practical solutions to mining challenges, reducing uncertainty around long term mine scheduling.
If you ask a geologist or scheduler how certain they are about their model or schedule, they might be confident or say ‘it’s economically viable’. But have you ever tried to quantify that certainty or uncertainty?
Reducing uncertainty around long term mine scheduling is the aim of a partnership with the University of Adelaide as part of a South Australian research and industry program.
The Uncertainty Modelling project uses several new technologies, the first being Maptek DomainMCF.
‘Using machine learning to predict geological domains is highly efficient, requiring little in the way of input parameters. This generates models that include a measure of uncertainty, which can then be used to make informed decisions,’ commented Steve Sullivan, Technical Lead for DomainMCF.
An aggregate model is generated by averaging multiple DomainMCF runs that naturally exhibit variability where the geology is uncertain. This aggregate model is used to create an ultimate pit and stage design and provide the basis for a long term schedule using Maptek Evolution Strategy.
Optimisation on the aggregate model creates a net-present-value-optimised strategic mine schedule. This is where conventional analysis stops. However, by evaluating the schedule on each run in turn, insight is gained into the economic impact of geological uncertainty.
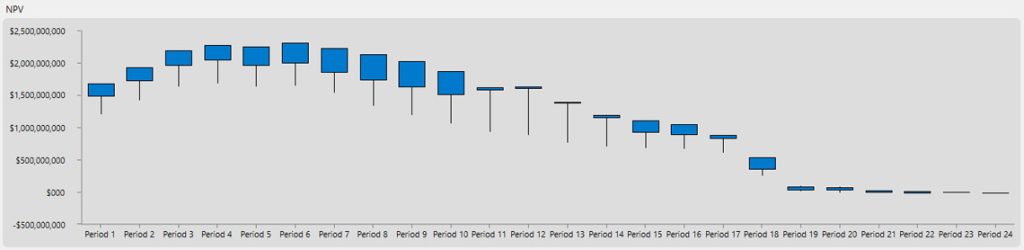
Ten runs from the same composite data using DomainMCF were processed through the Evolution Strategy optimisation engine. Preliminary results show that uncertainty in the geology can affect profit by up to $300M per period.
Schedules can be analysed to judge how sensitive a project is to changes in geological interpretation, an aspect that has been overlooked in the past.
This alone is a powerful decision making tool for drilling programs and pit design. An aim for the next stage of the project is to adjust the optimisation to reduce the gap between the best and worst result for each period. This should inherently reduce uncertainty and improve confidence in the result.
Dr Frank Neumann, Group leader for Optimisation and Logistics, and Dr Aneta Neumann from the University of Adelaide have been assisting with the uncertainty modelling project.
‘Mining involves a large variety of uncertainties that have significant impact on the profitability of an operation,’ said Dr Frank Neumann.
Profits achievable in different years are uncertain due to the quality or grade of ore mined. Constraints such as milling processes must resolve the stochastic and uncertain components.
‘Dealing with these stochastic components is crucial for an efficient and profitable operation,’ continued Neumann.
Stochastic constraints enable modelling of uncertainties, and limit failures or unwanted unprofitable events. This modelling can be achieved with machine learning techniques such as deep learning, which predicts outcomes based on previous learning.
After modelling the uncertainties, optimisation methods such as evolutionary computing in Evolution software can produce profitable plans that minimise the uncertainty around critical components.
Watch the video: Evolution Uncertainty modelling.
- A machine learning study showed that changes in geological interpretation can affect profit by up to $300M per period
- Ultimate pit and stage designs can be derived from aggregate models of geological uncertainty as the basis for a better long term schedule
- Optimisation techniques in Evolution can produce profitable plans that minimise uncertainty around critical components