June 2022 Issue Index
Improving on traditional modelling
A trial of Maptek DomainMCF at an underground metals mine concluded that machine learning will most likely become the preferred modelling method for mineral deposits.
Geologists at an underground mine in Western Australia have trialled Maptek DomainMCF machine learning for modelling their resource.
The IGO Limited Nova-Bollinger deposit is 700 km due east of Perth. The Nova operation mines and processes nickel-copper-cobalt sulphide ores.
Traditional resource modelling is based on a drillhole database containing 99 lithological and 11 sulphide mineralisation logging codes. From this database the mine geologists use implicit modelling to interpret 22 different domains – 21 sulphide domains and one all-encompassing waste halo domain.
The block modelling process is undertaken annually by a team of geologists on site and in the Perth corporate office, and takes several months to complete. They trialled DomainMCF in parallel to their standard workflow as part of the 2020 resource update.
The required inputs for DomainMCF are a csv file comprising drillhole database or composite data, and an optional upper and lower surface to define the spatial extents of the region to be modelled. A block model parameter file details the origin of the block model, the 3D spatial extents and the block/sub-block dimensions.
Grade estimates are done on a 6m x 6m x 2m block size and sub-blocks are permitted down to a quarter of the parent block size.
During the trial three primary tests were run using different versions of the drillhole file to explore the capabilities of the application and see how they compared to the existing workflow.
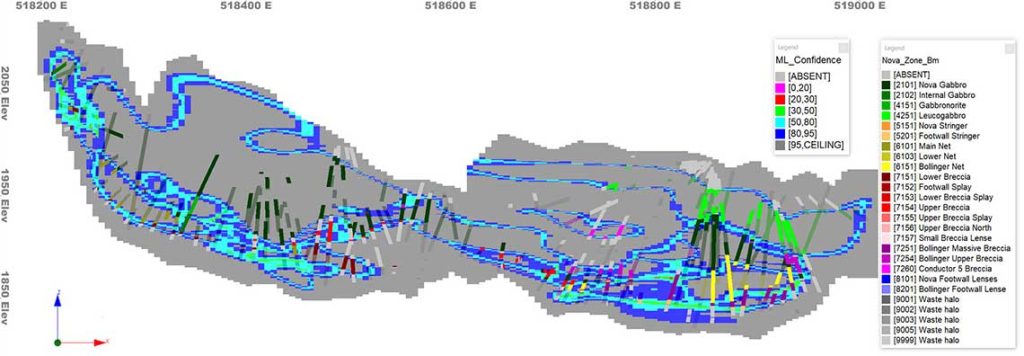
Test 1 provided DomainMCF with the drillhole composite file for the 22 different domains. Six chemical elements (Ni, Cu, Co, Fe, Mg and S) were provided to assist with the training phase of the machine learning algorithm.
For Test 2, the data used in the first test was augmented with lithology coded data from the drillhole information outside the estimation boundary limits. The chemical variables were again used to help train the algorithm.
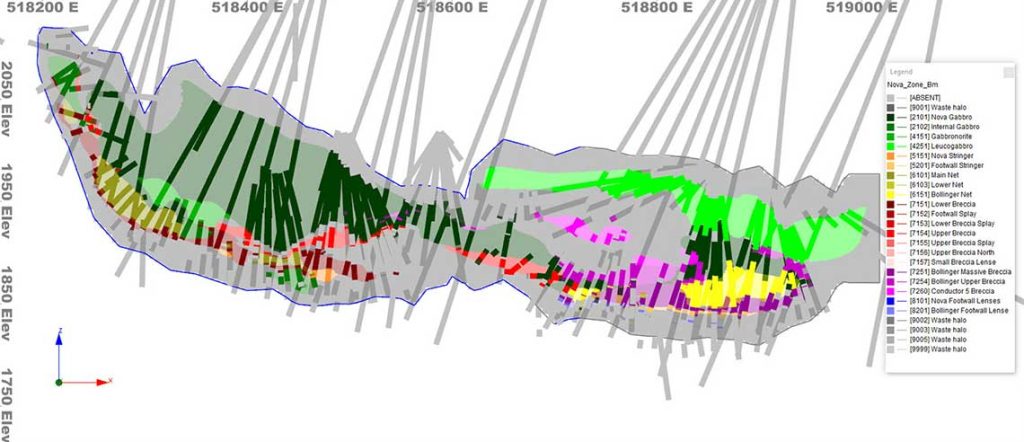
The purpose of this test was to determine if a combined sulphide and lithological model could be produced, and to see if giving DomainMCF additional information would impact the prediction of sulphide domains.
A hands off approach was used in Test 3 to see how DomainMCF modelled a file containing only mineralisation codes and the grouped lithology used for Test 2. None of the domain codes from Test 1 were used.
Test 3 examined if the DomainMCF model was comparable to a manually coded domain model and whether it was useful in the mineral resource estimate process.
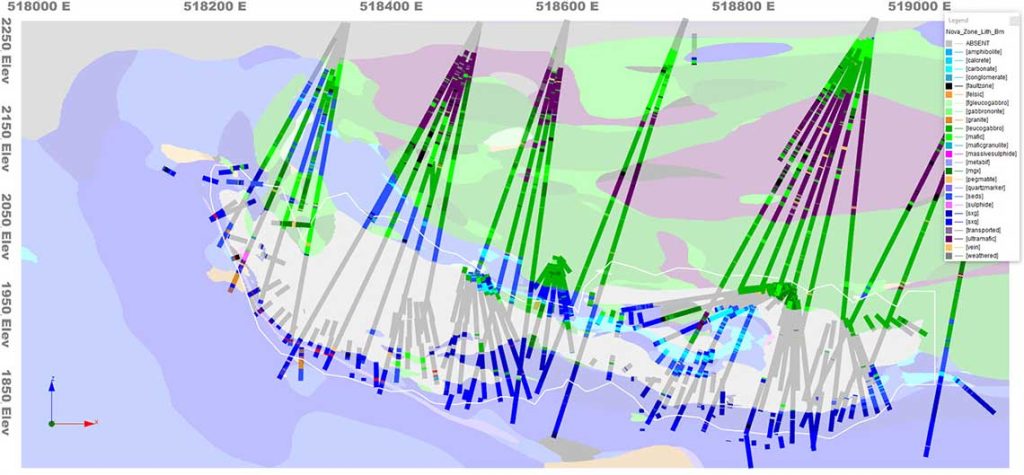
IGO Senior Mine Geologist Fletcher Pym presented the trial results in a paper to the AusIMM International Mining Geology Conference 2022.
‘We were able to run Test 3, which was a relatively complicated model, in 45 minutes,’ said Pym.
For Pym, Test 3 also showed that machine learning can produce very comprehensive models without the strong influence of a geologist.
Getting the data right from the start produces a high quality deposit model, which opens up new possibilities.
Because machine learning made resource modelling so much faster, senior staff had more time to focus on training less experienced core loggers. Improving the processes resulted in better quality drillhole logging.
‘Machine learning will become particularly attractive if the process can not only model geological domains, but also return reliable grade estimates for mine planning across the full range of mineralisation styles,’ said Pym.
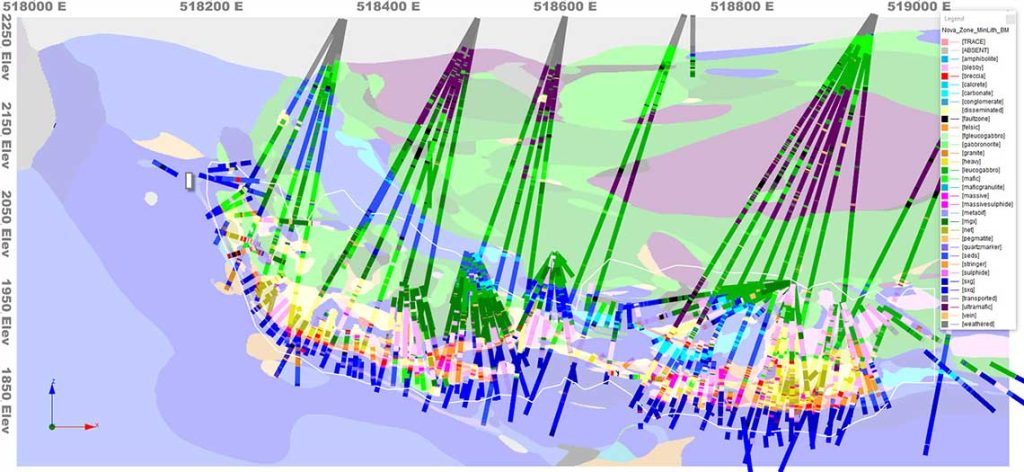
‘Providing a well-understood confidence measure can assist in risk quantification of both geology and grade.’
The study highlighted several advantages of machine learning:
- The inputs required for machine learning processing can be readily prepared in most resource modelling software.
- Machine learning modelling times are relatively short.
- The pay-by-use business model is more cost-effective than maintaining implicit modelling software systems.
- The machine learning model returns an objective measure of uncertainty in the geological model, which is likely to be useful in mineral resource classification and mining reconciliation work.
- Multiple different geological models can be prepared in parallel, meeting the JORC requirement to investigate ‘the effect, if any, of alternative interpretations on mineral resource estimation’.
Maptek Technical Lead for DomainMCF Steve Sullivan is excited at the potential of machine learning for revolutionising resource modelling.
‘I’m amazed at the response – we are already seeing companies subscribe to DomainMCF for use in domain modelling for their 2022 resource reports,’ said Sullivan.
‘Machine learning works best when all the available data is presented, as shown in Test 3. The more data the better.’
‘The industry is struggling to find experienced personnel during the current mining boom, so embedding years of experience into smart systems helps get the job done on time and under budget.’
Maptek continues to work on proposed enhancements following feedback from industry trials. Grade trend prediction was added in the March 2022 release and a strong uptake by customers will drive further advances.
Thanks to
Fletcher Pym
Senior Mine Geologist
IGO Limited
- Geologists compared machine learning and traditional approaches when preparing a resource update for the IGO Limited Nova-Bollinger underground mine
- Faster processing with DomainMCF meant senior staff had more time to focus on training less experienced core loggers
- Machine learning also delivers a well-understood confidence measure, which helps in risk quantification of both geology and grade