December 2020 Issue Index
Data driven geology
Technological advances have matured machine learning to a point where it can be readily and practically applied by solutions such as Maptek DomainMCF.
Maptek DomainMCF uses machine learning to translate geological data into resource models in dramatically less time than traditional methods. This approach is transforming the way we think about our data.
Digital transformation requires data analytics. To drive your operation from these analytics, you need to adopt a data driven culture. Not just you, your team or your site, but the entire company needs to embrace change to benefit from transition to digital systems.
This requires making systems which are robust, repeatable and independent of who operates them. It requires moving away from a traditional hierarchical way of working to a more distributed model. From a data point of view this can be termed the democratisation of data.
Democratisation of data
Maptek Senior Technical Sales Specialist and Technical Lead – DomainMCF, Steve Sullivan considers the changing nature of the workforce means this shift in mindset is critical to success.
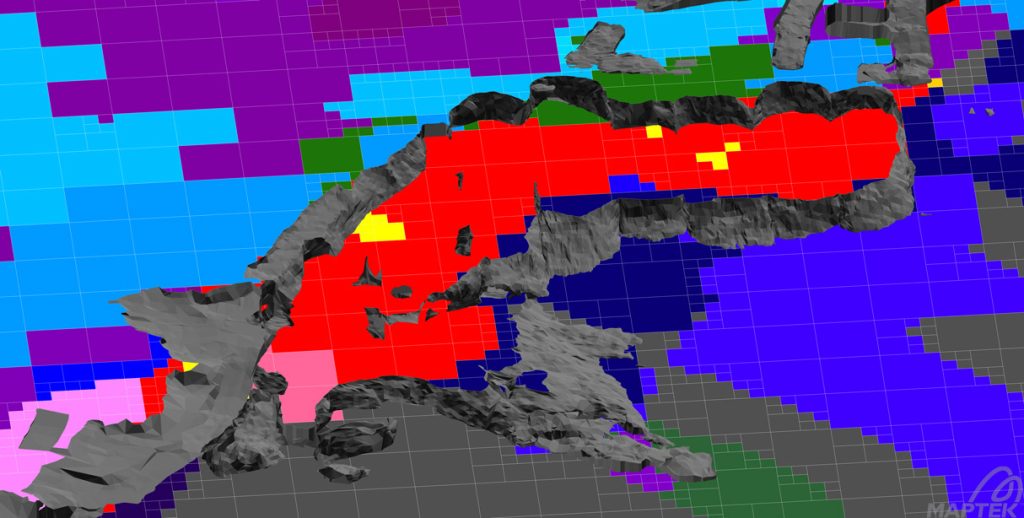
‘When I started my career in the 1980s at a smallish mine there were about five levels of geologist,’ Sullivan said.
‘That hierarchical structure has changed. With people moving around more we’ve lost continuity and consistency of thought.’
A data driven approach links and automates geological processes to provide an up-to-date single source of truth for an operation, liberating geologists to analyse the results.
Computers are now doing a lot of the back end and tedious work, which frees geologists up to do more analysis and higher level thinking.
This paradigm shift has been made possible by recent technological advances, particularly the maturation of machine learning to a point where it can be readily and practically applied by solutions such as DomainMCF.
Ten years ago geologists could still do all the geological processes manually but it would take days or weeks. People today don’t have the time to manually generate different scenarios to consider.
Machine learning techniques and fast computers help geologists come up with 10 scenarios within an hour, evaluate those scenarios and choose one that is optimal for their operation.
Cloud computing has also made this approach more practical as there is no need for the capital outlay or maintenance costs – companies can just pay for computer time.
Safety and economics
The key benefits of the data driven approach are safety and economics. Safety is always going to be the highest concern, but what does that really mean?
‘In any open cut mine, geology determines whether the walls will stay up, and underground whether drives will stay up, so there’s a huge safety imperative around understanding your geological structures,’ explained Sullivan.
‘The geology is unchanging, it’s our perception of the geology that changes as we get more information.’
Without proper knowledge of the uncertainty in a model, value can also often be over or underestimated.
In a recently reported case a $140 million dollar loss of value to the shareholders arose from overestimation.
A data driven approach removes much of the personal geological bias and makes for a consistent process.
Maptek stresses the importance of validating data and building interpretation parameters into the process that meet an operation’s unique requirements.
Every site has slightly different geology, so the architecture must match the individual workflows.
‘Preparing for a data driven approach requires thinking, planning and analysis – and time. Early adopters can reap the benefits of DomainMCF right now,’ concluded Sullivan.
- Digitalisation is the pathway to the democratisation of data, allowing organisations to realise the benefits of a continuously updated single source of truth
- A data-driven culture embraces systems which are robust, repeatable and user-independent, meeting the needs of a mobile, shift-based and geographically dispersed workforce
- Establishing data validation and interpretation regimes can help overcome personal biases when it comes to defining geological models